In this article
The value add of directly accessing de-identified clinical notes
The sequel’s better than the original: The next source of RWD
Painting the picture: Clinical notes in action
Meeting industry demands: Sample use cases to enhance research
Start exploring, extracting and learning with unstructured notes
The value add of directly accessing de-identified clinical notes
Claims and structured electronic health record (EHR) data are tried-and-true — perhaps even foundational — real-world data (RWD) sources. Life sciences researchers have trusted these RWD for years to support research and investigations across the product lifecycle, from development through post-marketing.
However good these data may be, they’re inherently selective. For example: What you see in clinical records or claims is limited by the information health care providers — and by extension billers and coders — input in pre-existing fields for reimbursement purposes or clinical care documentation. Some important, more descriptive information, such as location or severity of specific symptoms, may get lost in the shuffle.
For a good number of research studies, claims and EHR data do the trick. But what if you need a little bit more detail? Especially those details that are not material to reimbursement or justification of level of care delivered, and ultimately get left on the cutting room floor.
This is where clinician-captured notes present a great adjunct. By directly accessing de-identified clinical notes, you can get valuable additional insight beyond the purview of typical structured data fields.
The sequel’s better than the original: The next source of RWD
If you could access millions of de-identified clinician notes for, say, a diabetes cohort, what research questions would you ask? What new hypotheses would you test?
Clinical notes present an opportunity for do-it-yourself, flexible discovery. The notes themselves have been there in patient records all along, but because of recent advances in technology and investments, de-identified cohorts are now available for this deeper exploration.
Consider a diabetes cohort, for example. You could explore, annotate and extract details from the notes such as:
- Complications (retinopathy, congestive heart failure, etc.)
- Disease severity (mild, moderate, severe, complicated, advanced)
- Additional medications (insulin, GLP-1, etc.)
- Detailed physical findings such as early signs of vascular changes or symptoms of peripheral neuropathy
Deriving value from these data often goes hand-in-hand with natural language processing (NLP) and machine learning (ML) technology. By applying your organization’s own NLP or ML model to a cohort of unstructured notes, you can arm yourself with data outputs that are unique to your research needs.
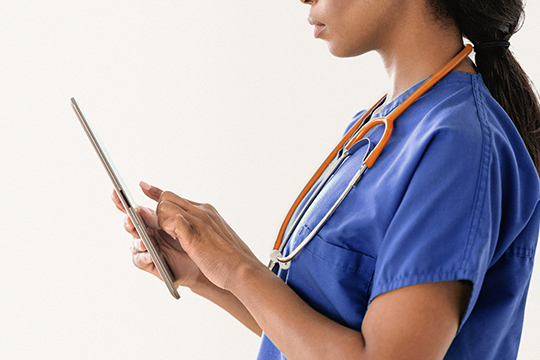
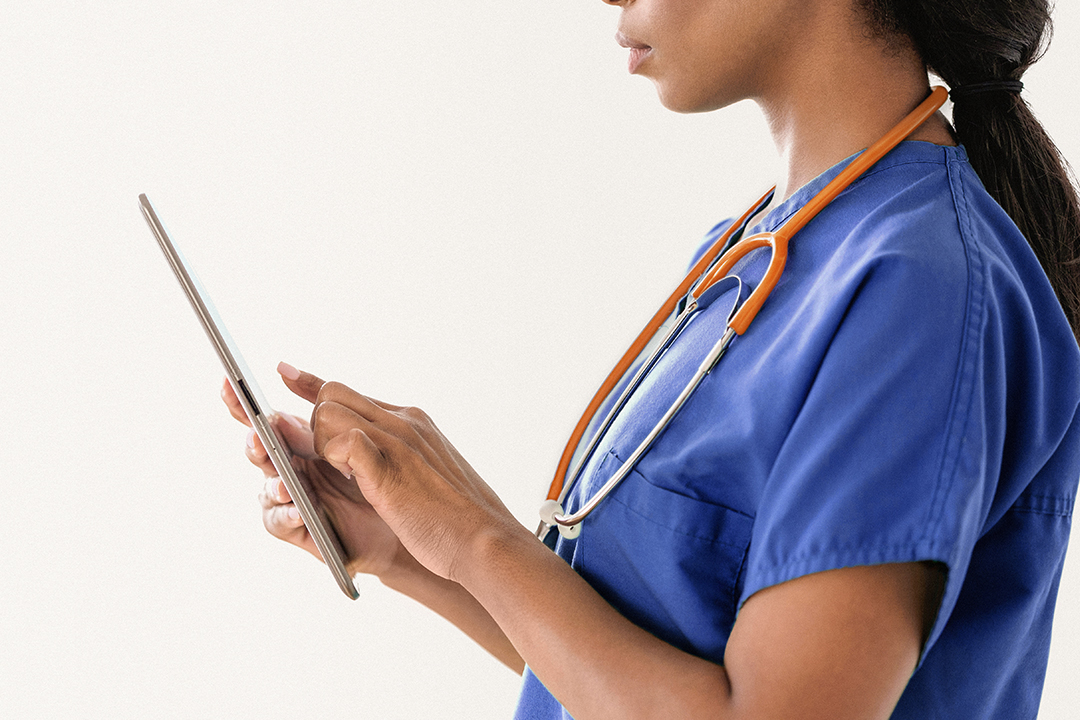
Painting the picture: Clinical notes in action
Bolster your research with richer details
In one recent study, Optum Life Sciences researchers assessed the cost differences, by severity of cognitive decline, in patients with dementia.* Cognitive assessment tools (CAT) scores were used to classify the severity of dementia.
The team first examined a small sample of notes that contained mentions of CAT, including their natural variations used by physicians. With direct access to the notes, researchers identified dozens of variations of CAT — such as mini-mental state exam, MMSE, Montreal cognitive assessment, MOCA exam — rather than relying on a single term in a pre-structured data field. The researchers were then able to manually identify patterns in the notes associated with mentions of CAT.
Finally, they applied NLP on the remaining notes to identify CAT scores. Out of the 101,126 patients identified, the researchers observed CAT scores in 3% and 9% of structured and unstructured data, respectively. The information the researchers needed was present in structured data — but only to a certain extent.
These findings show that unstructured notes captured 3 times as many test scores compared to structured data. That’s meaningful for multiple reasons:
- Demonstrates that structured data may be missing or underreporting utilization of important diagnostics.
- Provides researchers a larger pool of patients to work with when conducting analyses, increasing confidence in the data.
- Allows researchers to more accurately characterize patterns around how physicians are using these tests.
- Gives researchers the ability to better understand care and disease progression.
The study concluded that patients with higher scores, and therefore more severe disease, had higher average medical costs. This emphasizes the need for the earlier identification of these patients for more timely intervention to reduce disease burden and promote downstream cost savings.
Identify larger patient populations to uncover more information
In another example, Optum researchers partnered with a biopharma company to apply NLP to a random sampling of 1,000 clinical notes to identify and characterize patients with chronic cough. The team identified 4,818 patients with chronic cough, of which 37% were identified using NLP-identified cough mentions in clinical notes alone, compared to 16% by diagnosis codes and/or written medication orders. More than twice as many patients were identified in the notes versus structured data alone.
This study demonstrates how granular symptoms are more easily identified in notes versus structured data. For conditions that lack specific diagnosis codes, like chronic cough, clinical notes present an opportunity to better understand a specific patient population and select patients for future research studies.
Access to provider notes documenting care more holistically can improve patient characterization and provide more detailed observations, leading to enhanced takeaways and research.
Meeting industry demands: Sample use cases to enhance research
Consider how the following sample applications can help your team uncover new insights:
What can you do with clinical notes data? | How can you apply learnings from clinical notes data? |
---|---|
What can you do with clinical notes data?
Determine triggers in medication switching, factors in patient adherence and nonadherence | How can you apply learnings from clinical notes data?Understand the patient story underpinning treatment changes |
What can you do with clinical notes data? Monitor physician prescribing patterns around clinical events | How can you apply learnings from clinical notes data?Inform physician medical education strategies and assess the quality of care being provided to patients |
What can you do with clinical notes data? Discover patient cohorts for conditions not identified well or underrepresented in structured codes | How can you apply learnings from clinical notes data?Run studies focused on rare and underdiagnosed conditions |
What can you do with clinical notes data? Classify lifestyle status (e.g., physical activity, diet, etc.) of patients based on clinical outcomes | How can you apply learnings from clinical notes data?Develop detailed phenotypes to support the development and commercialization of products |
Evidently, the use cases are broad. Which is crucial, considering how demands from industry stakeholders continue to change. Plus, market factors — such as financial pressures to innovate due to rising costs, the desire to keep pace with NLP growth in the global market and evolving regulatory requirements — increase the need for data to generate robust evidence.
Clinical notes research enables richer insights across the patient care journey, improving hypotheses and the types of evidence generated in outcomes research.
Start exploring, extracting and learning with unstructured notes
Employing de-identified clinical notes can add a new layer of rigor and robustness to your research. Accessing notes can give you a glimpse into the world of patient-provider interactions that most structured data fields just don’t provide. And compliantly linking the outputs of notes extraction back to your structured EHR data can help close any remaining gaps in the patient journey.
Of course, working with clinical notes isn’t without its challenges. Each individual organization has their own level of comfortability with the NLP technology and clinical knowledge necessary to mine meaningful details from provider notes. But many organizations are already strategizing ways to get the talent and resources needed to incorporate emerging RWD sources in their research.
No matter what therapeutic area you’re working in, there’s an opportunity to deepen your understanding of how patients and health care providers behave in real-world care settings. Fuel your research with a more flexible and complex data source today.
Related content

Reconcile your RWD expectations to maximize your investment
Understand how routine clinical practice impacts information captured in real-world data (RWD).

6 guiding steps for selecting a fit-for-purpose data set
Using RWD that are not fit for purpose can waste time and money. Review 6 simple steps to guide your research towards the right data.
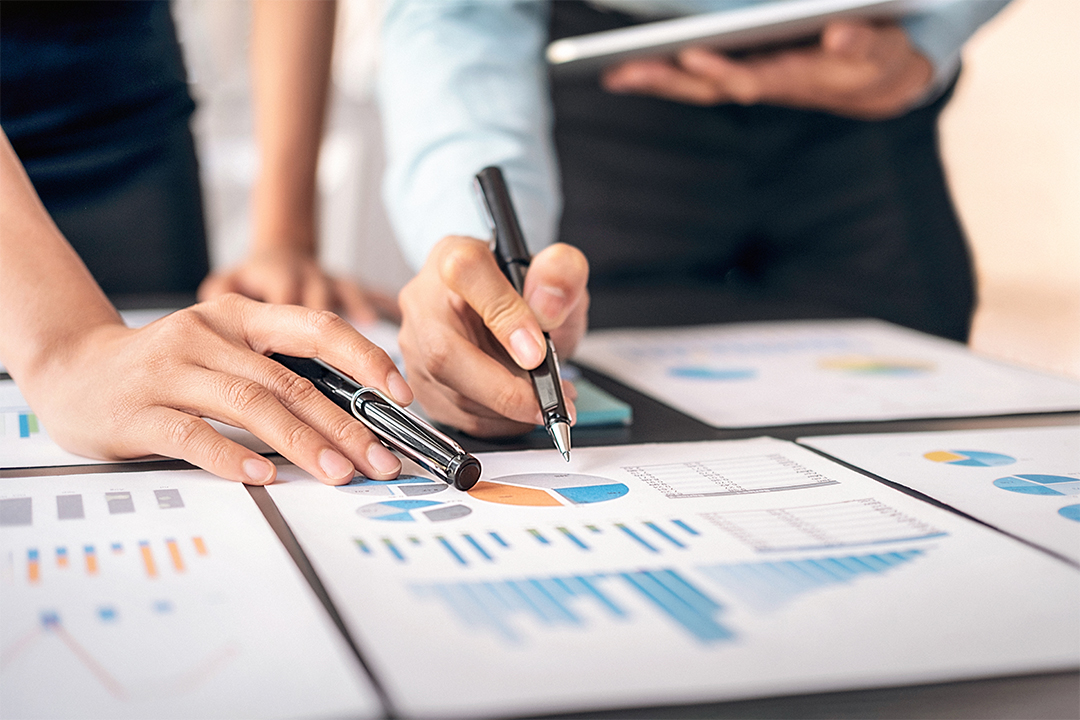
The 6 trends shaping pharma strategies in 2024
Life sciences market experts from Optum and Advisory Board share perspectives on trends that will influence the future drug value chain.
Sources
*Verma V, Rastogi M, Tara T, et al. Dementia: Uncovering Insights from Physician Notes using Cognitive Assessments. The Academy of Managed Care Pharmacy (AMCP). Apr 15–18, 2024. New Orleans, LA, USA. Presentation no F4.